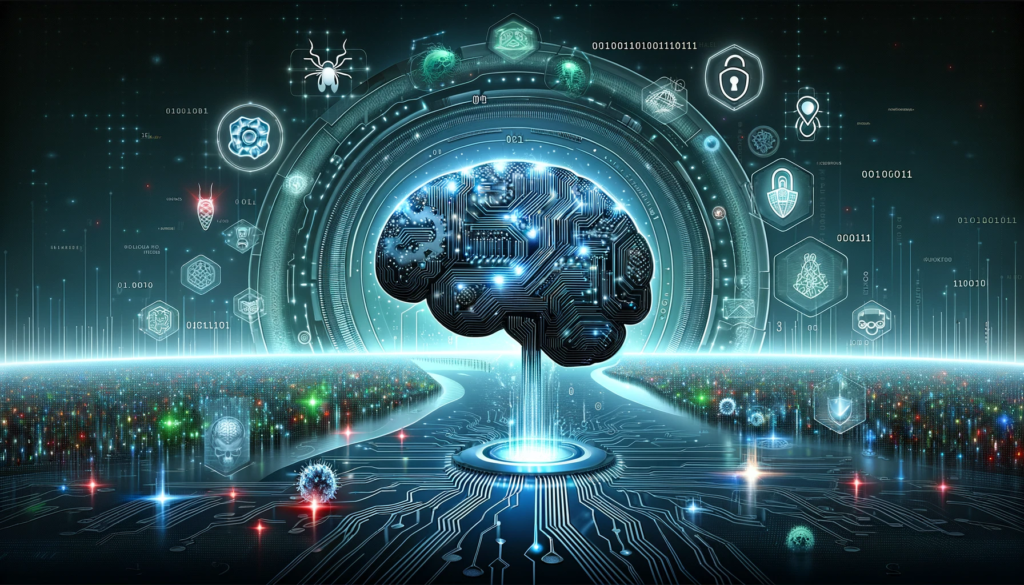
Machine learning (ML) is increasingly becoming an essential tool in cybersecurity due to the rapid digitization and growing complexity of cyber threats. Its applications in cybersecurity are diverse and crucial for addressing modern security challenges effectively.
Applications of Machine Learning in Cybersecurity
- Automated Glitch Detection: ML applications can rapidly identify irregular behaviors in systems, networks, or servers. This capability enables security teams to pinpoint the exact threat causing glitches, enhancing the responsiveness to potential security breaches.
- Zero-Day Attack Detection: ML advances beyond legacy security solutions by detecting multiple threats simultaneously, including previously unseen malware types. This capacity is critical for protecting organizations from potential zero-day attacks, which exploit unknown vulnerabilities.
- Automating Security Tasks: ML can automate laborious and repetitive tasks like malware analysis, network log analysis, and vulnerability assessments. This integration speeds up threat response and enables organizations to take immediate action against detected threats.
- Preventing SQL Injection-based Breaches: AI and ML help in preventing SQL injection attacks, a common technique used by attackers to infiltrate network systems. By learning the patterns of code modification, ML can identify and mitigate such breaches.
- Eliminating Compromised Password Attacks: Utilizing pattern-matching and constraint-based algorithms, ML aids in detecting threat patterns related to login systems, usernames, and passwords, enhancing the security of sensitive information.
- Combating Bot Attacks: ML is employed to control internet bots, which can be manipulated by attackers to infiltrate business networks. ML helps in distinguishing between genuine and malicious bot activities.
- Phishing Detection: Advanced ML algorithms improve the accuracy and speed of detecting phishing attempts by differentiating between malicious and harmless URLs, thereby identifying and mitigating phishing threats.
- Threat Categorization: ML analyzes large data sets related to security measures and tools, helping security teams classify threats based on severity. Indicators of Compromise (IOCs) are used to monitor, identify, and respond to threats efficiently.
Challenges in Applying Machine Learning to Cybersecurity
- Data Difficulties: One significant challenge is developing models using datasets with highly imbalanced distributions of target variables, which is common in cybersecurity applications like intrusion and malware detection.
- Higher Accuracy Requirements: Cybersecurity applications demand much higher accuracy from ML models, as even a small error rate in categorizing data packets can lead to severe consequences.
- Explainability of Models: Understanding the results of ML models is crucial for taking appropriate action in cybersecurity contexts.
- Talent Scarcity: The field requires professionals who possess both ML and cybersecurity expertise, a combination that is currently scarce.
- Securing ML Models: Ensuring the security of ML models and data used in cybersecurity is of paramount importance due to the sensitive nature of the data involved.
Machine learning is shaping the future of cybersecurity by offering sophisticated solutions to detect and respond to a wide array of cyber threats. However, leveraging ML in this domain also comes with unique challenges, such as data quality, model accuracy, and security, which need to be addressed to maximize its potential effectively.